Пресс-центр / новости / Наука /
Неоднородность ландшафта приспособленности GFP и дизайн белков на основе алгоритмов машинного обучения
Понимание взаимосвязи между генотипом и фенотипом — ландшафтом приспособленности — проясняет фундаментальные законы наследственности (Canale et al., 2018) и может в конечном итоге создать новые методы дизайна белков (Alley et al., 2019). Ландшафт приспособленности часто представляют в виде многомерной поверхности (Kondrashov and Kondrashov, 2015), одним измерением которой является приспособленность или фенотип, а другие измерения — локусы генотипа.
Несмотря на то, что ландшафты приспособленности некоторых белков были охарактеризованы экспериментально (Hartman and Tullman-Ercek 2019; Sarkisyan et al. 2016), подобные исследования больших белков все еще затруднены из-за огромного пространства возможных генотипов. Более того, характеристика таких ландшафтов приспособленности затруднена эпистатическими взаимодействиями между аминокислотами — зависимостью эффектов мутаций друг от друга, что является довольно частым явлением (Russ et al. 2020). Предсказание эпистаза на основании имеющихся данных является сложной задачей (Pokusaeva et al. 2019). Лучшим на сегодняшний день способом изучения ландшафтов приспособленности является направленная эволюция — экспериментальный подход, имитирующий естественный отбор и предполагающий случайное внесение мутаций в последовательность и получение множества мутантных форм (Chen et al. 2018). В качестве альтернативы используется рациональный подход к дизайну белков, при котором получают новые варианты на основе данных, полученных при изучении известных белковых структур (Anishchenko et al. 2021).
В настоящей работе международным коллективом с участим ученых из Группы синтетической биологии и Группы молекулярных меток для оптической наноскопии ИБХ РАН были использованы оба подхода для создания новых вариантов встречающихся в природе зеленых флуоресцентных белков путем получения десятков тысяч мутантных вариантов GFP и оценки их способности флуоресцировать (Рисунок 1). Кроме того, были использованы алгоритмы машинного обучения для предсказания функциональности других вариантов GFP и расширения ландшафта приспособленности зеленых флуоресцентных белков.
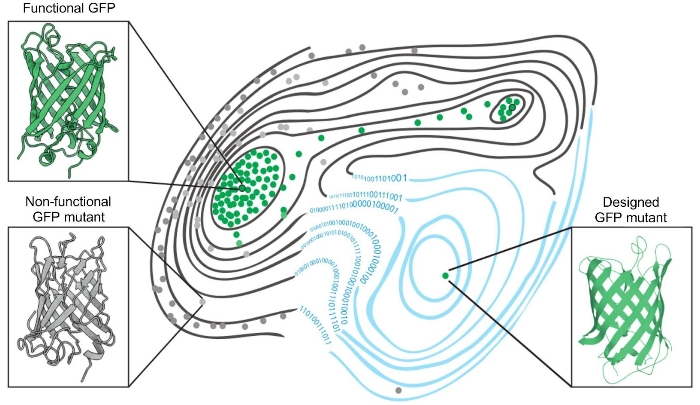
Рисунок 1. Ландшафт приспособленности зеленых флуоресцентных белков. Два встречающихся в природе зеленых флуоресцентных белка — зеленые точки, обведенные черным; функциональные мутантные белки, способные флуоресцировать — зеленые точки; нефункциональное варианты — серые точки. Применение алгоритма машинного обучения расширило ландшафт приспособленности (справа; синие контурные линии) за счет включения предсказанных мутаций, не проверенных экспериментально. Это привело к созданию функциональных, отличных от встречающихся в природе, синтетических вариантов (зеленая точка внизу справа), которые находятся на пиках ландшафта приспособленности.
Авторы смогли разработать светящийся вариант GFP, несущий 48 мутаций по сравнению с природными белками. Чтобы оценить, может ли разработанный алгоритм быть эффективно использован для других белков, авторы провели эксперименты с тремя GFP, которые произошли от эволюционно далеких видов — cgreGFP, amacGFP и ppluGFP2. Они обнаружили, что алгоритм на основе машинного обучения лучше предсказывает функциональные варианты cgreGFP, чем amacGFP и ppluGFP2. Анализ ландшафта приспособленности показал, что гомологи различались по количеству вносимых мутаций, при которых белки сохраняли активность: в среднем от трех до четырех мутаций для cgreGFP и avGFP и от семи до восьми мутаций в случае amacGFP и ppluGFP2. Белки также отличались общей устойчивостью: ppluGFP2 был стабилен при воздействии высоких температур, тогда как cgreGFP был более чувствителен к изменениям температуры. Повышенная мутационная чувствительность avGFP и cgreGFP, по-видимому, была обусловлена негативным эпистазом, при котором негативный эффект комбинации мутаций превышает эффект мутаций по отдельности.
В целом, опубликованные результаты показывают, что для работы алгоритма для создания функциональных вариантов белка и предсказания функции белка необходимы только данные об одиночных мутациях и их влиянии друг на друга (эпистаза низкого порядка). Это способствует развитию области белковой инженерии, поскольку это открытие предполагает, что предварительное знание об эпистазе высокого порядка — взаимодействия между большими наборами мутаций — не требуется для дизайна белков.
Работа опубликована в журнале eLife.
Список литературы
- Alley, Ethan C., Grigory Khimulya, Surojit Biswas, Mohammed AlQuraishi, and George M. Church. 2019. “Unified Rational Protein Engineering with Sequence-Based Deep Representation Learning.” Nature Methods 16 (12): 1315–22.
- Anishchenko, Ivan, Samuel J. Pellock, Tamuka M. Chidyausiku, Theresa A. Ramelot, Sergey Ovchinnikov, Jingzhou Hao, Khushboo Bafna, et al. 2021. “De Novo Protein Design by Deep Network Hallucination.” Nature 600 (7889): 547–52.
- Canale, Aneth S., Pamela A. Cote-Hammarlof, Julia M. Flynn, and Daniel Na Bolon. 2018. “Evolutionary Mechanisms Studied through Protein Fitness Landscapes.” Current Opinion in Structural Biology 48 (February): 141–48.
- Chen, Kai, Xiongyi Huang, S. B. Jennifer Kan, Ruijie K. Zhang, and Frances H. Arnold. 2018. “Enzymatic Construction of Highly Strained Carbocycles.” Science 360 (6384): 71–75.
- Hartman, Emily C., and Danielle Tullman-Ercek. 2019. “Learning from Protein Fitness Landscapes: A Review of Mutability, Epistasis, and Evolution.” Current Opinion in Systems Biology 14 (April): 25–31.
- Kondrashov, Dmitry A., and Fyodor A. Kondrashov. 2015. “Topological Features of Rugged Fitness Landscapes in Sequence Space.” Trends in Genetics: TIG 31 (1): 24–33.
- Pokusaeva, Victoria O., Dinara R. Usmanova, Ekaterina V. Putintseva, Lorena Espinar, Karen S. Sarkisyan, Alexander S. Mishin, Natalya S. Bogatyreva, et al. 2019. “An Experimental Assay of the Interactions of Amino Acids from Orthologous Sequences Shaping a Complex Fitness Landscape.” PLoS Genetics 15 (4): e1008079.
- Russ, William P., Matteo Figliuzzi, Christian Stocker, Pierre Barrat-Charlaix, Michael Socolich, Peter Kast, Donald Hilvert, et al. 2020. “An Evolution-Based Model for Designing Chorismate Mutase Enzymes.” Science 369 (6502): 440–45.
16 июня 2022 года